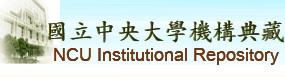 |
English
|
正體中文
|
简体中文
|
全文筆數/總筆數 : 80990/80990 (100%)
造訪人次 : 44990180
線上人數 : 4044
|
|
|
資料載入中.....
|
請使用永久網址來引用或連結此文件:
http://ir.lib.ncu.edu.tw/handle/987654321/92378
|
題名: | 使用深度卷積神經網路學習進行電阻率斷層掃描 (ERT) 解釋的觀測研究;Observational Study of the Electrical Resistivity Tomography (ERT) Interpretation Using Deep Convolutional Neural Network Learning |
作者: | 普古婉;Hiskiawan, Puguh |
貢獻者: | 地球科學學系 |
關鍵詞: | 電阻率斷層掃描 (ERT);卷積神經網絡 (CNN);深度學習;Wenner-Schlumberger;地球物理方法;Electrical Resistivity Tomography (ERT);Convolutional Neural Network (CNN);Deep Learning;Wenner-Schlumberger;Geophysical Methods |
日期: | 2024-01-15 |
上傳時間: | 2024-09-19 15:48:34 (UTC+8) |
出版者: | 國立中央大學 |
摘要: | 在本論文中,實施深度卷積神經網絡 (DCNN) 學習,為電阻率層析成像 (ERT) 處理數據提供另一種解釋。地球物理軟件專家對描繪反演電阻率剖面有一個共同的解釋。儘管如此,該解釋對地下的不確定性有一些限制,特別是在確定電阻率分佈的主要質量和顯示揭示的電阻率源方面。因此,觀察性研究旨在對短缺提供適當的解釋。 基於上述問題,本研究開發了幾種合成 ERT 模型,並使用具有局部降水引起的地下擾動的特定野外 ERT 數據集作為第一個觀測工作。在觀察到 [V/I] 處理之後,創建了輸入圖像。它們在 DCNN 學習中用於第二次觀察性研究是有形的。為了構建學習,使用了兩種架構技術:I 形 (ERT-INET) 和 U 形 (ERT-UNET) 。學習過程的最終結果是預測圖像。 Dropout 正則化提高了圖像產量。這些方法用於防止學習中的過度擬合。 成功的 DCNN 呈現了數據集的學習曲線。合成 ERT 模型的曲線結果表明準確度學習在 90% 以上,損失學習在 2% 以下;現場 ERT 揭示了大約 80% 的準確學習和低於 4% 的損失學習。一些統計評估已被用來評估學習表現;結果顯示相似的平均百分比。這項工作導致了預測圖像的開發,該圖像根據在自然地下檢查的類別產生一定程度的分類。 觀察工作的第三階段包括分類評估。接受者操作特徵 (ROC) 曲線用於比較兩種方案之間的預測圖像。該曲線能夠證明兩種架構之間的顯著差異。在三個電阻率類別中,混淆矩陣涵蓋了真實和預測的感知。觀察性研究的結果證明了兩種架構的成就。與這兩種架構一樣,合成 ERT 模型在所有類型的評估中都取得了 85% 以上的成績,包括現場 ERT。 本研究最後一項的觀察工作為綜合觀察,主要在於整合不同剖面產生的結果,也就是預測的反演剖面與實際剖面。結果顯示,ERT-UNET的性能較慢於其他方法。地球物理軟體專家生成反演剖面。一般性結論主要在地下大尺度構造及地球既有異常構造之揭示上發揮作用。這些方法更適用於ERT即時資料集及特定特徵的解釋方面。雖然這些發現可以被視為傳統地球物理方法的替代方案,但並不能替代傳統的地球物理軟體。 ;In this dissertation, deep convolutional neural network (DCNN) learning is implemented to provide an alternative interpretation for electrical resistivity tomography (ERT) processing data. Geophysical software experts have a common interpretation for delineating an inversion resistivity profile. Nonetheless, the interpretation has a few limitations on the uncertainty subsurface, particularly in determining the predominant quality of resistivity distributions and displaying a revealed resistivity source. As an outcome, the observational study aims to offer an appropriate interpretation of the shortages. Based on the aforementioned issues, this study developed several synthetic ERT models and used a specific field ERT dataset with subsurface perturbation due to local precipitation as the first observational work. Following observed [V/I] processing, an input image was created. They are tangible to use in DCNN learning for the second observational study. To construct the learning, two architecture techniques were used: I-shape (ERT-INET) and U-shape (ERT-UNET). The end result of the learning process is a predictive image. Dropout regularization improves image yield. The methods were used to prevent overfitting in the learning process. The successful DCNN presented a learning curve for the datasets. The curve results for synthetic ERT models indicated an accuracy learning above 90% and a loss learning below 2%; field ERT revealed an accuracy learning around 80% and a loss learning below 4%. Some statistical evaluations have been employed to evaluate learning performance, and the results showed a similar average percentage. This work has resulted in the development of a prediction image that yields a degree of classification based on the categories that were examined in the natural subsurface. The third phase of observational work included classification assessments. A receiver operating characteristic (ROC) curve was used to compare the predictive image between the two schemes. The curve was able to demonstrate the significant difference between the two architectures. In three resistivity categories, a confusion matrix covers true and predicted perceptions. The findings of the observational study demonstrated the accomplishments of two architectures. The synthetic ERT model, like both architectures, had achieved greater than 85% for all modules of assessments, including field ERT. The final piece of observational work for this study was a comprehensive observation, which was consolidating among profile yields, that is, prediction with inversion and ground truth profiles. The performance of the ERT-UNET is slower than the others. A geophysical software expert generates the inversion profiles. The general conclusions predicted the great subsurface and revealed the Earth′s existing anomalies. They are better suited for ERT real-time datasets, and the learning interpretations have particular features. While these discoveries can be considered an alternative approach to geophysical methods, the techniques cannot substitute traditional geophysical software. |
顯示於類別: | [Graduate Institute of Geophysics] Electronic Thesis & Dissertation
|
文件中的檔案:
檔案 |
描述 |
大小 | 格式 | 瀏覽次數 |
index.html | | 0Kb | HTML | 63 | 檢視/開啟 |
|
在NCUIR中所有的資料項目都受到原著作權保護.
|
::: Copyright National Central University. | 國立中央大學圖書館版權所有 | 收藏本站 | 設為首頁 | 最佳瀏覽畫面: 1024*768 | 建站日期:8-24-2009 :::